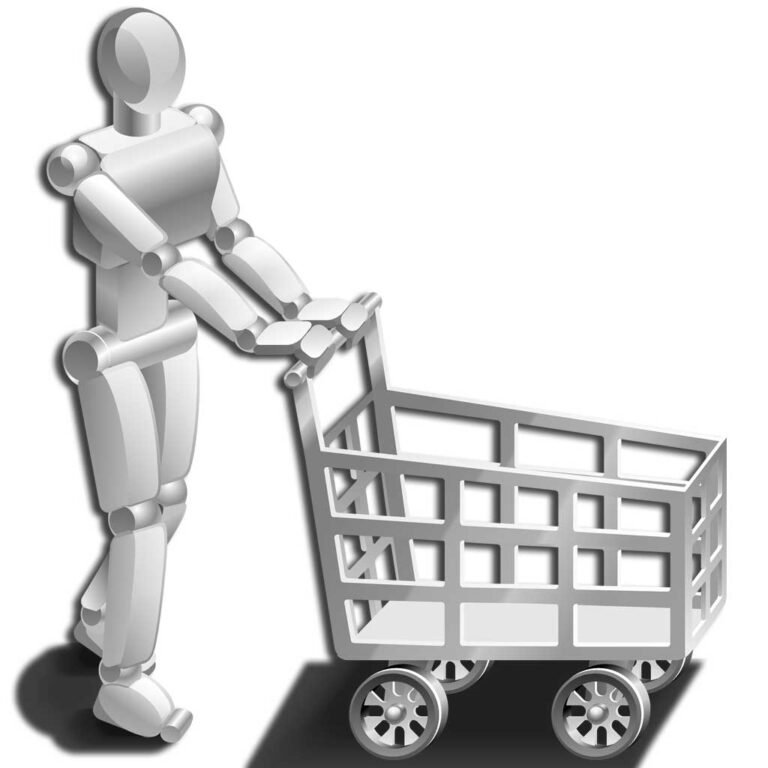
Leveraging Predictive Analytics in E-commerce for Enhanced User Experience
April 03, 2024 | Digital Techtune
E-commerce platforms are at the forefront of innovation, continually seeking ways to improve user experience (UX) and drive customer satisfaction. One of the most powerful tools in their arsenal is predictive analytics, which empowers these platforms to anticipate customer behavior, personalize interactions, optimize processes, and ultimately, boost sales and loyalty. This essay delves into the multifaceted realm of predictive analytics in e-commerce, exploring its applications, benefits, challenges, and future prospects.
Understanding Predictive Analytics in E-commerce
Predictive analytics involves the use of statistical algorithms, machine learning techniques, and data mining to analyze current and historical data, identify patterns, and forecast future trends or outcomes. In the context of e-commerce, predictive analytics leverages vast amounts of data, including customer demographics, purchase history, browsing behavior, geographic location, and even social media interactions, to anticipate consumer preferences and tailor offerings accordingly.
Enhancing User Experience through Personalization
Personalization lies at the heart of modern e-commerce, and predictive analytics plays a pivotal role in delivering personalized experiences to users. By analyzing past purchases, browsing patterns, and demographic information, e-commerce platforms can segment their audience and provide targeted product recommendations, tailored promotions, and customized content. This personalized approach not only improves user engagement but also increases the likelihood of conversion and repeat business.
For instance, platforms like Amazon and Netflix utilize sophisticated recommendation engines that leverage predictive analytics to suggest products or content based on a user’s past interactions and preferences. By analyzing historical data and employing algorithms such as collaborative filtering or content-based filtering, these platforms can predict what items a user is likely to be interested in, thereby enhancing the overall shopping or viewing experience.
Optimizing Inventory Management and Demand Forecasting
Predictive analytics also plays a crucial role in inventory management and demand forecasting for e-commerce businesses. By analyzing historical sales data, seasonality trends, market conditions, and even external factors like weather patterns, e-commerce platforms can predict future demand for specific products and optimize their inventory levels accordingly. This helps minimize stockouts, reduce overstocking, and improve overall supply chain efficiency.
Furthermore, predictive analytics enables e-commerce platforms to identify emerging trends and anticipate shifts in consumer preferences, allowing them to adapt their product offerings proactively. For example, a fashion retailer can use predictive analytics to forecast which styles or colors will be popular in the upcoming season, thereby informing their purchasing decisions and staying ahead of the competition.
Streamlining the Shopping Experience with Predictive Search and Navigation
Another area where predictive analytics can significantly enhance user experience is in search and navigation functionalities. By analyzing search queries, browsing behavior, and historical purchase data, e-commerce platforms can anticipate what products or categories a user is likely interested in and provide relevant suggestions in real-time. This predictive search capability not only speeds up the shopping process but also helps users discover new items they may not have otherwise found.
Moreover, predictive analytics can power intelligent filtering and sorting options, allowing users to refine their search results based on criteria such as price range, brand, size, or customer ratings. This enhances the usability of the platform and makes it easier for users to find exactly what they’re looking for, leading to higher satisfaction and conversion rates.
Improving Customer Service and Support
In addition to enhancing the shopping experience, predictive analytics can also improve customer service and support in e-commerce. By analyzing customer interactions, feedback, and sentiment data, platforms can anticipate potential issues or concerns before they escalate and proactively address them. For example, an e-commerce platform can use predictive analytics to identify customers who are likely to churn based on their recent behavior or purchase history and offer personalized incentives or support to retain them.
Furthermore, predictive analytics can power chatbots and virtual assistants that provide real-time assistance to users, answering their questions, resolving issues, and guiding them through the purchasing process. By leveraging natural language processing (NLP) and machine learning algorithms, these virtual assistants can understand user queries contextually and provide relevant and helpful responses, thereby enhancing the overall customer experience.
Challenges and Considerations
While predictive analytics offers tremendous potential for enhancing user experience in e-commerce, it also presents several challenges and considerations that businesses must address:
Data Availability & Quality:
Predictive analytics depands on heavily on the data quality and availability. E-commerce platforms must ensure that their data is accurate, comprehensive, and up-to-date to derive meaningful insights and predictions.
Privacy and Ethical Concerns:
The use of predictive analytics raises important privacy and ethical considerations, particularly concerning the collection and use of personal data. E-commerce platforms must be transparent about their data practices and prioritize user privacy and consent.
Algorithmic Bias:
Predictive analytics algorithms are not immune to bias, which can lead to unfair or discriminatory outcomes. E-commerce platforms must mitigate algorithmic bias by regularly auditing their algorithms, diversifying their data sources, and implementing fairness-aware machine learning techniques.
Integration and Implementation:
Implementing predictive analytics in e-commerce requires integration with existing systems and processes, which can be complex and resource-intensive. E-commerce platforms must invest in the necessary infrastructure, talent, and expertise to effectively deploy predictive analytics solutions.
Continuous Learning and Adaptation:
Predictive analytics is not a one-time endeavor but an ongoing process that requires continuous learning and adaptation. E-commerce platforms must monitor and evaluate the performance of their predictive models regularly and iterate based on feedback and new data.
Future Directions and Opportunities
Looking ahead, the future of predictive analytics in e-commerce looks promising, with several emerging trends and opportunities on the horizon:
AI-Powered Personalization:
Advancements in artificial intelligence (AI) and machine learning are enabling more sophisticated personalization techniques, such as hyper-personalization and real-time adaptation. E-commerce platforms can leverage AI to deliver highly personalized experiences tailored to individual preferences and behaviors.
Predictive Customer Lifetime Value (CLV) Modeling:
Predictive analytics can help e-commerce platforms forecast the lifetime value of individual customers, enabling them to prioritize high-value customers, tailor marketing strategies, and optimize retention efforts.
Augmented Reality (AR) and Virtual Reality (VR):
AR and VR technologies are reshaping the e-commerce landscape, offering immersive shopping experiences that bridge the gap between online and offline retail. Predictive analytics can enhance these experiences by personalizing virtual environments and suggesting relevant products based on user interactions.
Cross-Channel Integration:
As consumers increasingly engage with e-commerce platforms across multiple channels and devices, there is a growing need for seamless integration and consistency. Predictive analytics can help e-commerce platforms unify customer data from disparate sources and deliver cohesive experiences across channels.
Ethical AI and Responsible Data Practices:
With growing awareness of the ethical implications of AI and data-driven technologies, there is a growing emphasis on ethical AI and responsible data practices in e-commerce. Predictive analytics can be used to promote transparency, fairness, and accountability in algorithmic decision-making.
Conclusion
Predictive analytics holds immense potential for enhancing user experience in e-commerce, enabling platforms to deliver personalized recommendations, optimize inventory management, streamline the shopping process, improve customer service, and drive business growth. However, realizing this potential requires overcoming various challenges and considerations, including data quality, privacy, algorithmic bias, integration, and continuous learning. By addressing these challenges and embracing emerging trends and opportunities, e-commerce platforms can leverage predictive analytics to stay ahead of the curve and deliver compelling and differentiated experiences to their customers.