What are the latest trends in artificial intelligence?
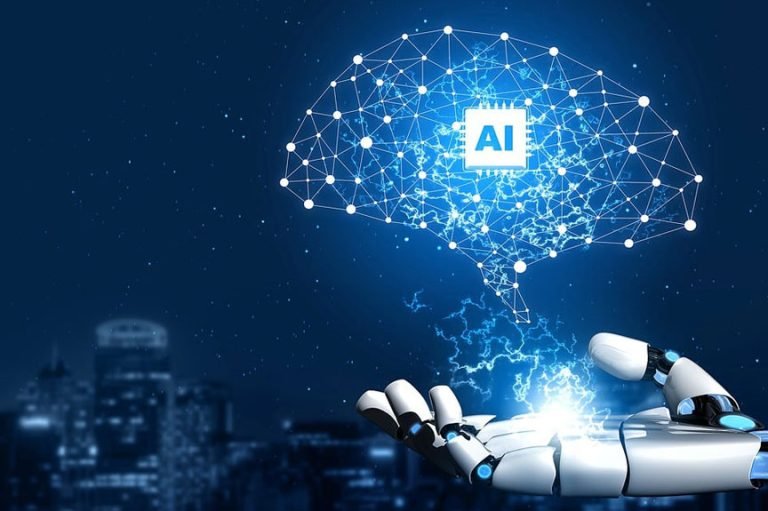
Many trends encompass different types of domains including computer versions, robotics, natural language processing, machine learning, and AI ethics. Below, we delve into some of the most prominent trends:
Deep Learning Advancements: Deep learning, a subset of machine learning, remains at the forefront of AI research. Recent trends focus on improving deep learning models’ efficiency, scalability, and interpretability. Techniques such as attention mechanisms, Transformers, and Capsule Networks have shown promise in enhancing model performance across diverse applications.
Generative Models: Generative models like Generative Adversarial Networks (GANs) and Variational Autoencoders (VAEs) have gained traction for their ability to create synthetic data, images, and even text. These models have applications in image generation, data augmentation, and content creation, among others.
Explainable AI (XAI): As AI systems become more complex, there’s a growing need for transparency and interpretability. XAI techniques aim to make AI models more understandable to humans by providing insights into model decisions. Methods such as LIME (Local Interpretable Model-Agnostic Explanations) and SHAP (SHapley Additive exPlanations) are gaining popularity in this space.
Edge AI: With the proliferation of Internet of Things (IoT) devices, there’s a shift towards deploying AI models directly on edge devices such as smartphones, cameras, and sensors. Edge AI enables real-time processing of data locally, reducing latency and enhancing privacy by minimizing data transfer to the cloud.
AI Ethics and Bias Mitigation: As AI systems impact various aspects of society, there’s growing awareness of the ethical implications and biases inherent in these systems. Efforts are underway to develop frameworks and tools for ensuring fairness, transparency, and accountability in AI algorithms and applications.
Federated Learning: Federated learning allows training machine learning models across decentralized devices while preserving data privacy. This approach is particularly useful in scenarios where data cannot be centralized due to privacy concerns or regulatory restrictions, such as healthcare and finance.
AI for Healthcare: AI for Healthcare: AI revolutionizes healthcare by enabling personalized treatment plans, early disease detection, and drug discovery. Recent trends include the use of AI for medical imaging analysis, predictive analytics for patient outcomes, and natural language processing for clinical documentation.
AI in Finance: In the finance sector, AI is employed for fraud detection, risk assessment, algorithmic trading, and customer service automation. Advanced AI techniques like reinforcement learning are being applied to optimize trading strategies and portfolio management.
AI in Autonomous Vehicles: Autonomous vehicles rely heavily on AI for perception, decision-making, and control. Recent trends in this field include the development of more robust perception algorithms, simulation-based training for reinforcement learning, and regulatory advancements to facilitate the deployment of autonomous vehicles.
AI Governance and Regulation: Governments and regulatory bodies are increasingly focusing on AI governance to address concerns related to data privacy, cybersecurity, and algorithmic accountability. Regulations such as the General Data Protection Regulation (GDPR) in Europe and the Algorithmic Accountability Act in the United States are examples of efforts to regulate AI use responsibly.
Conversational AI: Natural language processing (NLP) techniques have led to significant advancements in conversational AI, enabling virtual assistants, chatbots, and voice-enabled interfaces to interact with users more naturally. Trends include the development of multilingual models, emotion recognition, and context-aware dialogue systems.
AI in Agriculture: AI technologies are being applied in agriculture to optimize crop yields, monitor plant health, and automate tasks such as irrigation and harvesting. Innovations in computer vision, remote sensing, and predictive analytics are driving advancements in precision agriculture.
AI in Education: AI is transforming education through personalized learning experiences, adaptive tutoring systems, and automated grading. Trends include the use of AI for student assessment, content recommendation, and virtual classroom environments.
Quantum AI: While still in its infancy, the intersection of quantum computing and AI holds promise for solving complex optimization problems and accelerating machine learning algorithms. Quantum AI research focuses on developing quantum-enhanced machine learning models and algorithms.
AI Collaboration and Open Source: Collaboration and knowledge-sharing within the AI community are fostering innovation and accelerating research progress. Open-source frameworks like TensorFlow, PyTorch, and scikit-learn are driving accessibility and democratization of AI technologies.
In conclusion, the latest trends in artificial intelligence encompass a wide range of domains, from deep learning advancements and generative models to AI ethics, edge computing, and AI applications in various sectors such as healthcare, finance, and autonomous vehicles. As AI continues to evolve, it’s crucial to address ethical, regulatory, and societal implications while harnessing its transformative potential for the benefit of humanity.